OpenAI’s Secret Sauce? Millions of YouTube Hours Haunt GPT-4
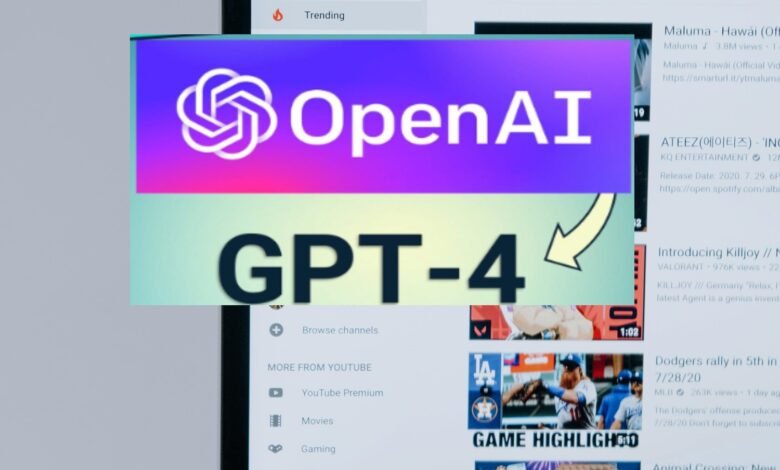
Introduction OpenAI’s Secret Sauce
Start with a captivating scenario where OpenAI’s Secret Sauce someone interacts with GPT-4, showcasing its remarkable capabilities. Briefly mention the mystery behind its impressive performance.
Announce OpenAI’s recent revelation about training GPT-4 on a massive dataset of YouTube videos, totaling millions of hours.
Highlight the growing unease surrounding this training method, raising questions about potential biases and ethical implications.
Understanding GPT-4: The Power of Large Language Models
What is GPT-4? Provide a clear explanation of Large Language Models (LLMs) like GPT-4, their basic functionalities, and their potential to revolutionize various fields.
The Power of Training Data: Discuss the crucial role of training data in shaping the capabilities and limitations of LLMs. Explain how vast amounts of data can enhance an LLM’s ability to generate human-quality text, translate languages, write different kinds of creative content, and answer questions in an informative way.
The US AI Race: Briefly mention the ongoing competition in Artificial Intelligence (AI) research within the US, highlighting the pressure to achieve breakthroughs and the potential national security implications.
Earlier this week, The Wall Street Journal reported that AI businesses were struggling to collect high-quality training data. Today, The New York Times documented some of the ways businesses have coped with this. Unsurprisingly, it entails engaging in activities that fall under the misty grey area of AI copyright law.
The story begins with OpenAI, which, desperate for training data, purportedly built its Whisper audio transcription algorithm to overcome the challenge, transcribing over a million hours of YouTube videos to train GPT-4, its most powerful large language model. According to The New York Times, the corporation was aware of the legal issues but believed it was fair usage.
YouTube: A Feeding Ground for GPT-4
A Mountain of Content: Describe the immense volume and variety of content available on YouTube, encompassing everything from educational videos to entertainment clips, music videos, and user-generated content.
The Learning Potential: Explain how this vast dataset provides a rich training ground for GPT-4, exposing it to diverse language patterns, cultural references, and human interactions. The Dark Side of YouTube: Discuss the potential downsides of using YouTube data for LLM training. This could include:
* Exposure to biases and misinformation prevalent on the platform
* Learning offensive or harmful language used in some videos
* Privacy concerns surrounding the use of potentially identifiable content
This week, YouTube CEO Neal Mohan expressed similar sentiments about OpenAI potentially using YouTube to train its Sora video-generating model. Bryant stated that Google employs “technical and legal measures” to prohibit such illegal use “when we have a clear legal or technical basis to do so.”
According to Times sources, Google also obtained transcripts from YouTube. Bryant stated that the company taught its models “on some YouTube content, in accordance with our agreements with YouTube creators.”
The Ethics Debate: Is OpenAI Going Too Far?
Bias Amplification: Explore the risk of perpetuating and amplifying existing biases present in YouTube content, potentially leading to discriminatory outputs from GPT-4. The Transparency Gap: Criticize OpenAI’s lack of transparency regarding the specific selection criteria used for the YouTube video dataset and the potential for cherry-picking content that aligns with their goals. Mention growing calls for stricter regulations governing the use of personal data in AI research, particularly concerning privacy and potential misuse.
Meta, too, encountered limitations in the availability of excellent training data, and in recordings obtained by the Times, its AI team discussed its unauthorized exploitation of copyrighted works while attempting to catch up to OpenAI. After reviewing “almost every English-language book, essay, poem, and news article available on the internet,” the corporation appears to have explored paying for book rights or even purchasing a major publisher entirely. It was also reportedly hampered in its ability to use consumer data as a result of privacy-focused reforms implemented in the aftermath of the Cambridge Analytica debacle.
Potential Benefits vs. Risks: Weigh the potential benefits of using large datasets like YouTube videos for LLM training against the identified ethical risks and privacy concerns.
The Quest for Mitigations: Discuss potential solutions and mitigation strategies that OpenAI and other AI research institutions could implement to address the ethical concerns surrounding data collection and training methods. This could include:
* Anonymizing user data before using it for training
* Developing filtering mechanisms to exclude harmful or biased content
* Implementing stricter ethical guidelines and oversight for AI research projects
The Importance of Public Discourse: Emphasize the need for open and transparent communication between AI researchers, policymakers, and the public to ensure responsible development and deployment of LLMs like GPT-4.
Google, OpenAI, and the rest of the AI training community are dealing with rapidly depleting training data for their models, which improve as more data is absorbed. The Journal reported last week that companies may outperform new content by 2028.
Possible solutions to the problem mentioned by the Journal on Monday include training models on “synthetic” data generated by their own models or so-called “curriculum learning,” which involves feeding models high-quality data in an ordered fashion in the hopes that they can make “smarter connections between concepts” using far less information, but neither approach has been proven. However, the corporations’ other choice is to use everything they can find, whether they have permission or not, and based on many cases brought in the last year or so, that route is, let’s say, more than a little risky.
Conclusion
The Future of AI: Express a hopeful outlook for the future of AI research, emphasizing the need for a balanced approach that prioritizes innovation alongside ethical considerations and safeguards user privacy.
A Call to Action: End with a call to action, urging readers to stay informed about AI advancements, participate in discussions about responsible AI development, and hold research institutions accountable for ethical practices.
Your point of view caught my eye and was very interesting. Thanks.